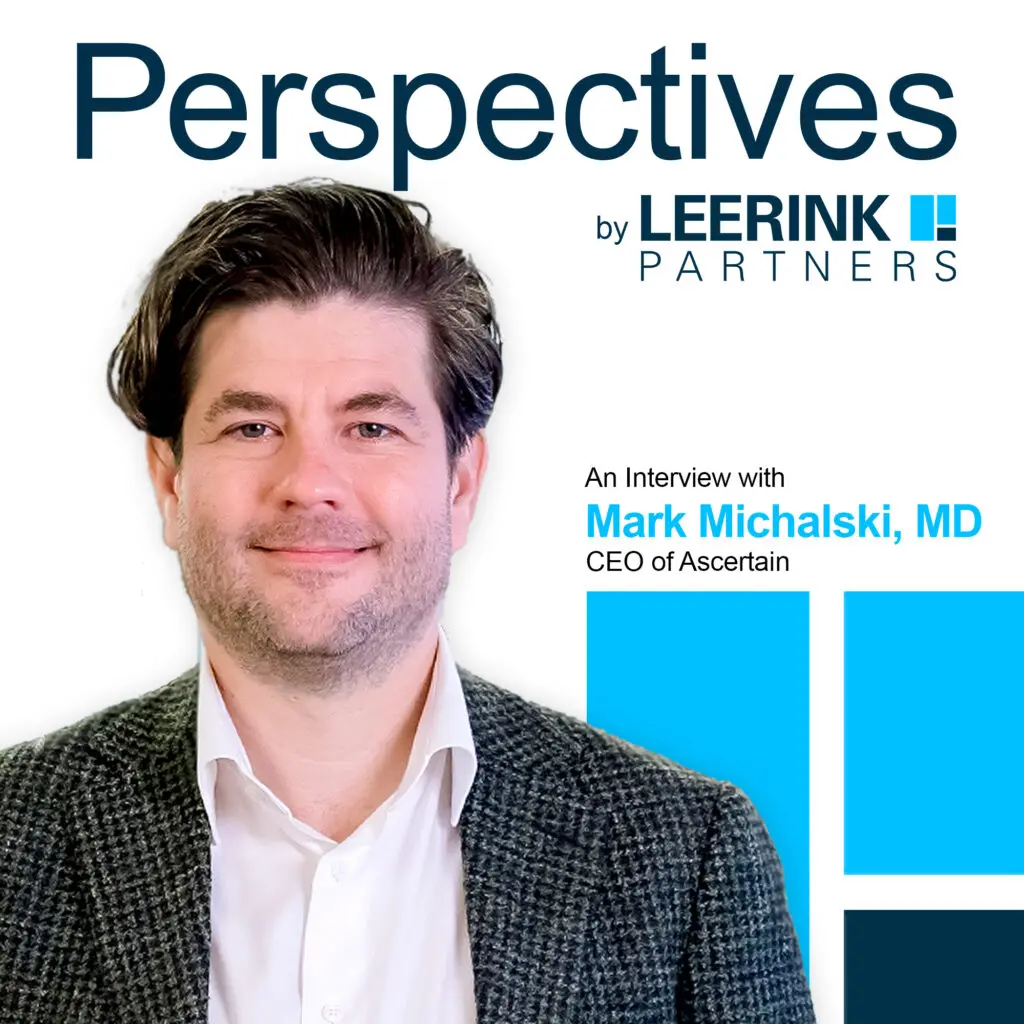
March 12, 2024
Welcome to Perspectives, Leerink Partners’ signature podcast, where we share our insights and interview leaders across the industry to get their perspective on how they’re driving innovation. We’ll also be digging into the backstory to learn more about what has most influenced their success. Be sure to check out all episodes by Leerink Partners.
Thad Davis: Hi, my name is Thad Davis. I’m a senior managing director here at Leerink Partners, and I’m joined here today by Mark, the CEO, my friend, and leader of Ascertain. Mark, thanks for joining the podcast today.
Mark Michalski, MD: Yeah, thanks for having me, Thad. It’s good to see you.
Thad Davis: Good to see you. Like we were saying to see you a little bit more over the next week or so. So, any of listeners that are out of ViVE, you might run into Mark or myself at ViVe. Mark, why don’t you give the audience an understanding of kind of many of the other guess probably people have high familiarity with. But I think you’re here to educate a little bit on Ascertain and what you’re up to. So just give us a summary.
Mark Michalski, MD: Thanks Thad. I’m the CEO of Ascertain and our mission is to bring best in class automation tech to healthcare enterprise operations. I spent some time at Amazon. I saw how there are a lot of industries that had benefited from the adoption of automation, supply chain and manufacturing and fulfillment for global internet retail brand. But in healthcare, we haven’t really done that very effectively.
Thad Davis: Whatcha talking about? What are you kidding me? Everything’s working great. I don’t know why. I don’t even know why we’re having this podcast. Yeah, yeah.
Mark Michalski, MD: It’s tough. We’ve gotten very, very good at making sure that you have your air fryer to you in two days, but we haven’t really figured out how to deliver care to you during the worst day of your life. Bringing some of that technology to healthcare and healthcare operations is where we’re focused. That’s what Ascertain is about and happy to talk more about it.
Thad Davis: We’ll unpack that because I think that there’s a lot of very interesting vectors and angles around Ascertain, which I think many people are trying to tackle this problem. I think there’s well positioned companies and not so well positioned companies, and I think Ascertain is probably on the well positioned side. You’ve been spending time around your whole career, spending time around the intersection of medical technology, data science devices, and clinical medicine. I think that’s probably, in my view here at Ascertain the pinnacle of a summary of the career. This is the end game around the unification of those areas, which seems to be a big theme throughout your life and career.
Mark Michalski, MD: My mother could probably tell you I have a hard time focusing. I moved from one thing to the other, but I think in this case it might’ve been beneficial. It’s what it means to do data science and healthcare these days. It’s more than just understanding how to roll an LLM or something like train an LLM or roll a rag model or something like that. It’s understanding the context of that data and where it comes from, how it’s generated. That’s where typically you see whether these systems work or not. And it’s not super surprising if you think about it. My text messages to my friends are probably make no sense outside of context. We have all these inside jokes, but if you have that context, you can make sense of it. Same with my inbox, my email, and it’s not really any different with healthcare information. Back when I was doing stuff in radiology, we found that computer vision algorithms, they really changed their performance changes based on the type of scanner that you’re using, the manufacturer for an MRI, what kind of sequence that you were deploying. And it’s the same thing here with gen AI. I think having that contextual awareness is really important. Long story short, I think having people at that intersection is really key.
Thad Davis: I think that a lot of folks attacking the same area you’re attacking here lack the broad scope context that I think a little bit of your background provides. But you originally grew up in the OC, so LA area. Tell me about the early years. What young Mark like?
Mark Michalski, MD: Young Mark was into science fiction, Legos, was not a very good student.
Thad Davis: You and I grew up the same way. I had a four by three by two-foot box filled with Legos to all the sets that had torn down. I would rebuild and then have the tube on literally tube TV on the background with Star Trek on.
Mark Michalski, MD: Exactly. Star Trek, the next generation was definitely influenced there. Born, raised about five minutes from the beach in Huntington Beach, and then we went to South County. I think one of the things was I was always into building stuff. That’s true. And again, not a super good student. My mother was a school teacher, and so to her dismay, I wasn’t a bad student, but it was hard to get me engaged. And that was all through high school. I did music and things like that. But I’m lucky that the community college system in California is so good because I really didn’t find my legs until much later.
Thad Davis: The UCLA exit, you didn’t enter as a freshman to UCLA?
Mark Michalski, MD: No, I was a transfer student. Yeah, I was a transfer student.
Thad Davis: Oh wow. Okay. So basically, you were like, you found religion, you found educational or religion later.
Mark Michalski, MD: I got a D in my first calculus class. It was interesting for me because I was also dealing with just a smidge of dyslexia, which I think is another thing. So, I just wasn’t a real good student, but I think it was when I entered community college and I started to recognize that I didn’t want to stay. I loved staying near the beach and surfing. I’m a poor surfer, but I love it. But I didn’t want to do that for the rest of my life. And so, I was lucky to get transferred to UCLA and there was something about being able to be able to try different things without the heat of being at a major university. In my early years, I was just a late bloomer. I went to UCLA because this degree program, they don’t do it anymore, but it’s cybernetics.
Thad Davis: Yeah, I looked that up. I was like, cybernetics. And I read that basically it was an interdisciplinary program. There’s only been what, a few hundred people that have passed through this degree over the years. I don’t know if you’ve seen the, Northwestern has the IDS program, which is very similar to that. And I was like, oh, which very limited amount of IDS people at Northwestern. I thought that was amazing. I was like, “oh, that sounds so cool.”
Mark Michalski, MD: That’s why I went, how am I not going to do the cybernetics? And to make it even better is because it was the University of California, the governor’s signatures on all the diplomas, and Arnold Schwarzenegger was the governor at the time.
Thad Davis: Oh, you were like, “I have a cybernetics degree. You’re like cyber system, with the T 800 assigned, signed to my diploma.” This is perfect.
Mark Michalski, MD: Yeah, yeah. No, it was perfect. And I think it all comes together. I was bad student probably because I didn’t like to listen to my teachers and I did cybernetics primarily. I don’t want anyone tell me what to study and let me do all sorts of stuff. Anyway, I think I ended up doing, it’s basically electrical engineering and computer science, all neuroscience, and that set me up really nicely for Stanford.
Thad Davis: You went from UCLA up to NorCal. Now granted, I think that probably the latter half of your career as we get into it, does not necessarily say traditional surgical medicine or anything like that. But how did you leap from going to an interdisciplinary near engineering style program into medicine of all things?
Mark Michalski, MD: Partly was I started getting involved in the children’s hospital at UCLA and after understanding that at Mattel, the Children’s Hospital, I started to recognize that the stakes on this stuff was pretty high. Could do some very interesting engineering just for the sake of engineering Legos for the sake of Legos, right? But it felt like there were high stakes for people, and as you’re entering college and starting to see the world outside yourself, I think that was eye-opening for me. And then part of the reason I went to Stanford was because I saw how technology was making some big impacts. One of the things I did while I was at UCLA is I set up a wireless network in Nepal back when there wasn’t a whole lot of, there weren’t internet connections out there and things like that. It was super cool.
Thad Davis: That’s an amazing thing to do. And also probably actually in all things, you could be in a political prison somewhere right now for setting up a WIFI network.
Mark Michalski, MD: Yeah we set up these wireless, wireless routers all around the Annapurna region. It was beautiful to help ’em with commerce. But yeah, it was in the middle of a Maoist revolution. We got taxed a couple times. But I think it was really in college when I started to recognize that this technology could really impact lives. And so that gave me a little bit more purpose, and that was what led me to Silicon Valley and why I decided to go to medical school there. And once you land in Silicon Valley for when I was there right after the bubble.
Thad Davis: This would’ve been early tens?
Mark Michalski, MD: Yeah, early tens. Yeah, early tens
Thad Davis: 2001, 2, 3, The aughts, was just talking to somebody. People don’t fully appreciate that. The post.com bubble in the valley. I spent a bunch of time pre banking in that sector, but it was one of the largest sectoral depressions of all time. It was like 25% of the valley was unemployed. There was like out of great pain becomes great art, so to speak. And there is a lot of just, I can’t even imagine being there during that time. The level of creativity would’ve been, or just problem solving or fix it, just expansive commercial thinking.
Mark Michalski, MD: It’s a really good point. Out of the bubble, there were a lot of people who needed to do something new who were brilliant, but it was also the rise of some of the tech giants that we all know and may love today. I had a roommate while I was in medical school who was working at Google, and he was adjacent to some of their really initial healthcare things. And I remember thinking to myself, those guys are doing at least as interesting stuff as we’re doing over at the medical school in healthcare. I got to understand that a little bit better.
Thad Davis: You spent a little bit of time hanging out there for just a brief spell, right?
Mark Michalski, MD: Yeah, yeah. For a short time, I worked for DotOrg, which a philanthropic brand. Interesting enough, it was under Larry Brilliant, who has got an incredible story. He wanted to do prediction and prevention of infectious diseases that are emerging into the human population. Just turns out he was probably at least a decade too early. So that program got shut down, but they ended up doing some really interesting things like predicting flu spikes before they entered the population, before the CDC could find them based on the search queries from the Google search stream. And just that really opened up a lot of eyes, I think mine included to what tech could do in healthcare.
Thad Davis: Yeah, it’s like use of data science, use of data exhaust use of things like that. Yeah.
Mark Michalski, MD: But I think at the almost exact same time, it demonstrated for the first time that tech was not very good at this. It was health faults and Google Health’s first attempt, which didn’t work out. So, I think that we started to get the first inclinations that the move fast break things, virtues at tech just sometimes didn’t apply in healthcare.
Thad Davis: Was there a heavy-duty monolithic system already in place where things happen and people are used to interfacing with that, and people don’t necessarily adopt new in healthcare, even if it’s the coolest thing ever. Also, the third point I’ll make is coming back to what you said earlier, context is everything. A lot of tombstones in that graveyard of innovation around lack of context in healthcare.
Mark Michalski, MD: 100%. it could have worked, but I think now within the years, in retrospect, those initial forays into healthcare from the tech enterprises, I think they were important. But it’s still taken many cycles for big tech to find their way into healthcare. And it’s arguable how much they’re able to do it now, but I think we’re starting to see signals that they’re starting to find their footing.
Thad Davis: The bones are starting to appear of after a billion dollars of investment. You went to med school, and you went down the radiology route, which is obviously, I think at hindsight people would be like, “oh, of course radiology. That area makes sense. Kind of where Mark ended versus where he started from.” But at the time, how did you be like, “I’m going to be a radiologist, let’s go do that.”
Mark Michalski, MD: Oh gosh, I so many. I really had a lot of challenges deciding which way to go after medical school because nothing felt like it fit. And there were definitely moments when I decided I’m not going to do this medicine thing. This was interesting. We wanted to do something else, and radiology ended up being fit because I think some people go into radiology because they really like technology and they like healthcare, and they’d love to see the marriage of that. That’s why I went, and it turns out I was going into radiology because I thought it was the engineering discipline of medicine. But it turns out it’s more like art appreciation right now in that it’s like you people sort of get a sense of there’s an indistinct opacity in the right lower lobe, and this may be pneumonia. Some radiologists over time have gotten very good at turning that into really actionable data. But for me, I was always a little confused about how different radiology reads could actually end up ending up putting patients in different positions, even though it’s the same scan. This was one of those things that I loved the discipline, I loved the field. I also felt like it was time to bring some of that precision to the field. So, I was just very lucky that I was thinking that. The same thought that I think many people have had. I just happened to be lucky enough to be asking that question at the time when computer vision started taking up with deep learning around 2012.
Thad Davis: When were you doing your residency at Yale?
Mark Michalski, MD: 2011 to 2015 and 2015 and I was doing a PhD program there too. Didn’t quite get the PhD done.
Thad Davis: So for the folks that don’t understand, you were a Holman fellow, which within the American Board of Radiology, a specific designation around not necessarily clinical practice, but more research track radiology, if I’m not mistaken.
Mark Michalski, MD: That’s exactly right. Yeah. Because I was principally in radiology to build discover. That’s kind of how I oriented my residency.
Thad Davis: Also an appreciation that 11 to 15 timeframe is if you’re a radiology resident at that time, that’s also the rise during that time, if people probably don’t look back on it. But AI and machine learning sort of in healthcare was born heavily in the radiology area. There was a tremendous amount of investment during that time. There was a lot of teleradiology platforms. The services side of radiology began to consolidate as docs were like, “whoa, independent radiology needs to consolidate. There’s a storm ruin in radiology”, and then a ton of startups, but broad based AI startups, and then people trying to find targeted things, IBM must alone must have spent a few hundred million trying to pursue radiology machine and machine learning AI or machine learning radiology at the time around that to varying degrees of success over the years in those areas. But leaping from what you were saying there. You’re like, “wait a second, I’m around this research side. I’m seeing this transformation.” You spent a little bit of time with a few device companies, which were innovative devices, but ultimately this led you up to the mass general situation. What was the transition between the practice and then the Mass Gen thing?
Mark Michalski, MD: Deep learning was starting to take off, and that was really what we currently know of as the AI revolution was really built in computer vision. It was ImageNet, it was in 2012 when that all started. And then in radiology, we started to see the first start companies around 2015 or so, and I was working at two device companies, Butterfly and Hyperfine. One of the components of that, those technologies that they were imaging devices was using cough net, using deep learning to classify is this tumor, is this left ventricle? What’s the ejection fraction calculated automatically? And those sorts of things. And it became pretty clear pretty quickly that this was just going to change everything very quickly.
Thad Davis: Butterfly is a handheld ultrasonic device. So, you just hold that to somebody, and you have a phone next to you and the phone just goes, “here’s the ejection fraction”, which ejection fraction for those at home is the amount of force coming out of the heart on a pump. So basically, it’s a force measure of the heart.
Mark Michalski, MD: The whole thing around Butterfly was we were trying to reduce the cost to ultrasound so everyone could use it, but the problem isn’t really the cost. The problem is it’s hard to use ultrasound, it’s hard to figure out how to use that thing. And it was interesting because it was clear that the technology was moving so fast that if we wrote RO1s or Standard NIH grants, we would be left in the dust. So, what we did instead is we worked with industry to facilitate the development of these models and in partnership with them start to build these products because it just the cycle times and the grants, whereas just too long. So, we were able to scale that team pretty aggressively, especially for an academic enterprise.
Thad Davis: Using basically the purpose of the lab was, is that you had access to the the data, you had access to the oil reserves, so to speak, and then you began to do some exploratory drilling, as they would say in the energy sector. And then towards the tail end of that, then that’s when you became sort of connected with Amazon, who is expanding. Were you working with Amazon a lot at that point and they’re just like, “Hey, Mark, what are you doing there?”
Mark Michalski, MD: I think at that point, so before the CCDS at the Center for Clinical Data Science at Mass General, I had done my startup, those two startups, Butterfly, Hyperfine and my residency, and I was kind of ready to not be running it at that pace anymore. And I think Amazon gave me an opportunity to step back, learn, grow, actually have kind of an adult day job.
Thad David: You’re like, “I don’t want to be a privateer anymore. I just want to go in the Army. I want to go in the Army and have ’em give me orders so I don’t need to be a privateer.”
Mark Michalski, MD: Exactly. People in startups, I think we oftentimes think about work-life balance as phases of your life and the order of years as opposed to having your weekends off. So, it was a cushy job, but it was definitely a moment to sit back and reflect.
Thad Davis: If you look at your LinkedIn profile and you look at this flow, this actually stands out. It’s not that people would say, “oh, how does he end up at Amazon?” Obviously if you listened to this thread, it makes sense that you would be there. But Amazon is actually a relative detour in sort of the flow, because this is the first kind of time, the adult job where you had, this is an advisory type, this is a services thing. You’re taking your knowledge and helping others build versus everything before. Everything after has been focused on problem solving, creation. Not everybody can flip back and forth between I have been of service, I will be of service mode and then to I will create, creation will be had. How does that feel between those two worlds for you?
Mark Michalski, MD: It was really an exercise of understanding the problems that enterprises like the payers, the providers, what are the similarities, what are the differences? Where are they going? From a technology lens, they’ll oftentimes talk about how in the provider world, it’s oftentimes hard to even to get an insight about how much foundational work needs to happen within a healthcare enterprise, much less how do you bring in machine learning or advanced analytics. And so, getting a pulse on where the industry is, it’s really valuable. And I also think that for me, understanding how a hyperscaler does this kind of work.
Thad Davis: So now let’s focus on ascertain, and can you just take a minute for the listeners here and talk about the Aegis ventures, Northwell and Ascertain? Just describe the triangle there and who created what the involvement of the parties are, et cetera.
Mark Michalski, MD: Yeah, so Aegis is essentially a startup venture fund venture incubator that started out with some minority investments with Northwell that went successfully. And through that built a lot of trust. And around 2022, 2023, Aegis and Northwell decided that they wanted to play some big bets in AI. Aegis committed a hundred million to do this kind of work with Northwell in artificial intelligence and launched a couple joint ventures. And I was an advisor with Aegis I at the time, and we identified a couple opportunities for both Northwell and Aegis where they could really make some really fortuitous bets in the space. After a little bit of diving, I think it became clear that what they really were looking for was they were looking for some leadership to bring some of these technologies and some of these companies to the market. It became clear that this was an opportunity that I might want to do. We couldn’t find someone better to do it. Ascertain is one of those joint ventures, and it’s focused principally on those nonclinical operational inefficiencies that we have in the hospital. Stepping back, I work with the alumni association for my medical school, and recently I saw some statistics about how many of us are still in the practice of medicine in my class. And it’s pretty mind blowing. It’s probably around, it’s not quite half are no longer practicing. And Stanford’s kind of a unique place, it tends to create researchers. But I would say that for me, this speaks to how challenging it is to be a provider these days. Medicine’s a great gig if you take away all the paperwork that we have to do, all the overhead from the business perspective, it’s a wonderful thing to do. But I can tell you that the incentives are such that we’re pushing some very bright people out of medicine and into other industries. And I would probably say that all of us, no matter what’s going to happen with artificial intelligence, we’re all going to want a doctor that is empathetic and smart, even if they’re backed up by AI and ML when we’re sick, we’re going to want that. I can guarantee you every last one of us, that’s what we’re going to want. They may be using AI to make the diagnosis. They may be using advanced technologies to come up with personalized therapeutics, but they’re going to want a human who’s empathetic and smart. I want to get to a place where providers are taking care of patients and the bots are taking care of all the rest, because I think that’s where we all want to be. When we went to medical school, a lot of us were nursing school or PA school. That was the vision that we came with is we knew a lot about science, technology maybe. But ultimately, we all went there because we wanted to take care of people. And to the extent that we can return that vision, it’s interesting. You might need a very advanced technology to actually come up with a very retro view of what it is to practice medicine, a patient and a doctor together in a room talking about their medical issues.
Thad Davis: That’s the thing about healthcare. Everybody’s like, “it needs to be simpler.” That ship sailed. Simpler requires tearing the entire system down. That’s probably not happening. That’s not going to work. If we take what we have in front of us and begin to do work around that, what can we do smarter? We’re all smart people right here. What can we do with AI? Towards that, I think I had the fortune of getting a demo of some of the ongoing, and it’s both the prototype and some of the ongoing implementation you’re doing at Northwell. And I thought that your approach in kind of breaking down some of these very complex processes into multiple AI agents was a refreshing approach from a lot of the sort of more monolithic AI that was done maybe three years ago. And then I’ve actually reflected on taking this story out. I saw this demo and people are like, “oh, yeah, yeah, they’re doing it right”, and things like that. So maybe just that’s more of a comment, but what are your thoughts on the approach and how you’re getting practical engagement at the clinical level within Northwell?
Mark Michalski, MD: Yeah, this all stems from the way that we envision artificial intelligence being used here is it’s really enabling the best people within the practice and multiplying them and their capability. If you build it that way, then a lot of things then will fall out from that assumption. That is, you’ll want to create a system that’s very intuitive that aligns with the way that people currently interact with the world. And how do case managers and social workers do the process of getting a prior authorization for discharge to a skilled nursing facility or long-term care facility? They interact with a number of different stakeholders, and they manage them in different ways. So that’s the way that we set up our framework. The Ascertain framework is, as you said, it’s a set of AI agents that work for a shared goal, but all have different specialties. And that allows your case manager, your care worker, to understand when these systems need to be corrected, you know where that problem is intuitively because it’s that agent that say it needs to update its rules or the diagnosis code is wrong. You can update that. And it’s also very resilient. It’s just like a team of people if one fails, and the others sometimes can pick up the slack. There’s a couple reasons to do it. It makes it very intuitive; it makes it resilient, it makes it auditable, it makes it correctable.
Thad Davis: If you give a task too large to a person, because these LLM models approximate how people effectively think themselves. So, if you give a task that’s too multivariate, too large to a person, they might get it done, but you’re going to be like, “why’d you make all these decisions?” “I don’t know. I did it the way I thought it was” versus breaking the task down into three components. And then each person goes, “you go, well, why did you do your part a certain way?” And they go, “oh, well, I’ve learned a lot and I know this very narrow area. I don’t know what Johnny Q over there is doing, but I know what I did and here’s why.” And you go “no, you can’t do that. You have to do it this way because Johnny Q needs it that way”. “Oh, okay, I won’t do that anymore.” You’re like, perfect.
Mark Michalski, MD: Exactly. I also feel like it’s generalizable, right? So ultimately the question becomes what are the boundaries of what this kind of system can do? And at least for us, so RCM is chockfull of opportunities, the call centers, supply chain procurement, and then beyond. I am pretty convinced that the next couple years, just like most of the implementations of artificial intelligence for the last 10 years, there’ll be a couple bubbles and stumbles, but this in particular, this tech just having lived with cough nets and radiology and NLP, this is creepy how effective it works in our hands right now. So, it’s going to be an interesting couple of years.
Thad Davis: Yeah, exactly. I think this is one of these things like substantive productivity enhancements going forward here we’re just the beginning of massive productivity enhancement. Around, it is not necessarily, I think that we were talking about this a few weeks ago. This doesn’t have to be viewed through, people automatically, humans, they drift to the negative lens on this, and I’m not sure I’m going to go down the hardcore route of being a Silicon Valley AI apologist, but it can be viewed as, well, wait a second, these people could actually just do their jobs better and be happier for it and get more productivity done and be less frustrated at their job. That sounds pretty good. You’re like, “yeah, that sounds pretty good.” And the amount of power that would be required, especially in healthcare where the complexity is level 10 out of 10 versus auto reliability, but it is better to, the near to medium terms is productivity expediency versus necessarily replacement, which is just haven’t, at least from my own take, I haven’t seen a healthcare AI company where I’m like, oh, that’s going to replace a lot of people. I haven’t seen that yet.
Mark Michalski, MD: I don’t think that’s where we’re headed. Most of the, we look at how that’s implemented in different fields. It tends to converge on copilot, whether you’re a programmer, a lawyer,
Thad Davis: I would love to have a day-to-day copilot. There you go. I would love to have a machine that’s set there. I’m like, maybe it’s maybe going back to the beginning, it was like it just Star Trek enough. I would love just to ask the computer. I just wanted the computer to be my friend. I was like, I could be so much more productive and happy if I just had a copilot to help me do my job. What you were showing me in some of your processes. I was like, oh, no problem. You just show this to the person doing the job. They’ll be like, “yeah, I’ll take it. That sounds great. I’ll do that right now. I can go home at five versus six”. “Yeah, you can.” “You’re like, sounds good. Put it in.”
Mark Michalski, MD: Yeah. The existential stuff that people ask when these tools actually, when you’ve got some familiarity with them, you see what they can do. They do have limitations and where your place is and the universe alongside the technology, it just becomes another tool. But I got to tell you, it’s a really powerful tool. And I think now we’re at a place where really the failure modes no longer so much are in the technology world. It’s now very squarely. And how are we as people going to manage the change management aspect and the adoption of it, the education component of it, people who are training right now and advanced degrees in medicine and others practice is just going to be so different. And this goes from doctors through case managers, through nurses, through PAs, every one of ’em. This technology is really going to shift. So, I would say the next phase of innovation, maybe human innovation, how do you train, how do people interface with this effectively? The technology is going to be able to automate a lot.
Thad Davis: Yeah, completely agree. Well, besides thinking about how to implement models to improve efficiency in clinical gains in healthcare, surfing, are you surfing a lot anymore? What occupies your time besides a hundred hours a week at a startup?
Mark Michalski, MD: I am in New York. I know that Long Island’s got some surf, I haven’t gotten out there yet. Most of what I do to decompress is I’ll watch old music videos. I’m an old millennial, so I remember MTV and I’ll listen to Radiohead, watch Karma Police. That music video is just, it’s a work of art. That’s my problem-solving technique. I play music videos in the background.
Thad Davis: What’s one perspective that you are like, man, I wish I could pass along If I was a general healthcare person listening to this or just somebody who was like, wow, that’s an incredibly impressive career lifestyle. What would be one piece of advice you’d pass along?
Mark Michalski, MD: I’m a terrible surfer, but one of the things that’s very, that’s clear for me is you can only surf when the waves are out. Really. It’s nice to be out in the water, but if you want to surf, you got to go when the waves are, and in your career, there’s a couple of moments when the waves are out, like really out. Right now, it would be easy to dismiss gen AI or artificial intelligence as hype, excitement. But I think the waves are out, and I think it’s worth wherever you are, whatever you’re doing, I think it’s worth taking a look. How can you surf this wave right now? And it doesn’t mean learning how to build models, and it doesn’t mean jumping off and doing a startup. What it might just mean is how does this technology change your workflow and your practice? And I don’t think you can ignore it. This one. I think it’s one of those things where it’s worth learning. It’s worth figuring out how you can change your own workflows, your own orientation to what you do. This is a moment that’s pretty big, I think for all us.
Thad Davis: I agree with you kind of coming back to what we were talking about, the post dot com sectoral depression. I remember that time people were literally, after that, people were like, “yeah, the internet was a fad.” And I was like, “yeah, the internet no going to be like it. You’re just going to look up stuff online.” What is that? I think you’re missing the boat here has the same feel like no really does. This is fundamentally different. This is where we’re going. Mark, I appreciate you taking the time to drop by and have a conversation, and hopefully people find, I’m sure people find this super interesting. I think what you’re doing at Ascertain is just incredible and we wish you the best of luck over there.
Mark Michalski, MD: Thank you so much for having me.
Thank you for listening to this episode of our Perspectives podcast. If you are interested in participating in future podcasts or would like to learn more about Leerink Partners, please email us at info@leerink.com.